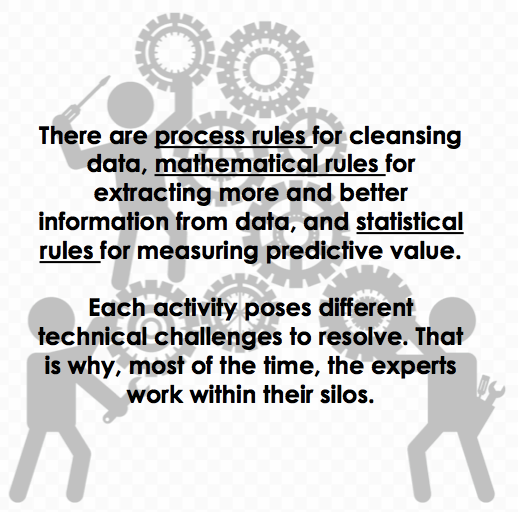
13 Sep Data vs. Predictive vs. Junk Science
Last week, a press release announced the launch of a credit scoring application of AI to natural-language processing and news media text analytics. Reading it, I wondered how many people had the confidence in their judgment to parse the offering. Just because a tool uses AI doesn’t make it intelligent.
Predictive credit analytics are complex. They are assembled in a sequence of activities involving data processing, statistical inference, financial modeling and standardized scoring. The informal assembly line spans three highly specialized knowledge siloes with big blind spots in-between. Paradoxically, the blind spots arise from the very deep, detailed knowledge subject matter experts have about properties of objects in that domain. “The devil’s in the details,” we say.
Experts keep the devils of production at bay. But very few experts are capable of high functioning outside their primary domain. In most business contexts, no natural oversight function will necessarily be in place to ensure continuous application of quality standards. Lack of oversight can lead to quality degradation as critical details slip between the assembly line cracks.
For example, quality standards in data management are related to storage, access, sharing, quantity, quality, security, and other properties that stabilize and enrich the data offering. But in the context of preparing the data for use in financial analysis, not all the data fields will be equally powerful and some may be unusable in their raw form. The same data will enter a different culture and be operated on by a new set of experts, statisticians, who are interested in different properties of the data and speak a different “language,” to raise the business value of the unstructured data to augment the structured data, of direct use in valuation.
As the data move further up the value chain, between statistical transformation of the data and financial modeling, they encounter another disconnect. Standing outside the process, this disconnect may appear trivial since commonplace statistical routines are used in financial modeling, like Excel canned statistical functions or Monte Carlo VBA macros. The disconnect is not trivial but treacherous. The ad hoc use of statistical add-ins is not a replacement for statistical reasoning. For, contrary to the claims of academic finance, financial modeling is not about mathematics. It is applied game theory. There is a fundamental tension between the objective representation of value using data and statistics, and creating a structure of payoffs to make money, which is the goal of financial modeling.
A naive consumer of credit analytics may not appreciate that most of the predictive value of predictive credit analytics is related to the quality of the second step, statistical inference. For cost management and claims validation reasons, it can be the most inconvenient step for a firm to support for commercialization–and it is the hardest for consumers to critique.
But predictive credit analytics are the future of capital allocation. The question of capital allocation intersects all human conversations, not only about power and wealth but also justice, equity, inclusion and growth. The same institutions that brought us credit models that “powered” the GFC have taken upon themselves a manifest destiny to drive their business towards every facet of our life, liberty and our pursuit of happiness. It remains an open question whether they perceive any fiduciary responsibility to the public.
In the same way that we view ourselves as agents in the world, we are also cogs in the giants’ credit machines. It is not too late to achieve a more equitable convergence between our choices and economic reason…for now. That is why I believe we all need to get smarter about how credit models work, and not simply “trust the experts.”